Have you ever wondered about the invisible ties that connect people on social media networks or in real life? One important fact is that not all links between individuals are easy to see, creating social networks with unobserved links. These connections can often lead to meaningful intergenerational connections that enrich our understanding of relationships.
This article will guide you through how network analysis and statistical modeling can help uncover these hidden connections, enhancing our understanding of interpersonal relationships and social interactions.
Get ready for an intriguing journey!
What are Social Networks?
Social networks are interconnected groups of people or organizations that interact through various relationships. They play a crucial role in shaping social structure and community dynamics.
Definition and examples
Social networks consist of nodes representing individuals or entities and links that show interpersonal relationships between them. For example, in a school network, students are nodes, and their friendships are links.
These connections form patterns that can be visually represented through graphs. Networks can vary widely; some have everyone connected to everyone else within certain groups, assuming equal linkage across the entire network.
Studying social networks requires accurate data collection and inference methods to identify both observed and unobserved connections. Techniques like link prediction help fill gaps by estimating missing relations based on existing data. Additionally, effective privacy settings management is essential to protect users' information while analyzing these networks.
In scenarios involving small numbers of individuals or incomplete information, statistical modeling becomes crucial for understanding the structure and dynamics of social interactions, including various factors affecting salary.
This approach enables researchers to explore relationship modeling, node prediction, and the overall impact of unobserved links on network estimation and social interaction modeling efficiently.
Importance of studying social networks
Understanding social networks is crucial for analyzing and predicting human behavior, information flow, and decision-making processes within groups. By studying social networks, including emerging snapchat trends, researchers can gain insights into how individuals form connections, share ideas, and influence one another.
This understanding is essential for various fields such as public health, marketing strategies, and organizational management. Moreover, the ability to infer social networks from observed groups provides valuable data for estimating network structures and accurately identifying missing links. Additionally, many people are turning to free streaming platforms for entertainment and information, which can also influence social interactions.
Through statistical modeling techniques and data inference methods in network modeling using graph theory principles, researchers can uncover unobserved relationships that significantly impact the dynamics of social networks.
Studying social networks also allows us to analyze the spread of ideas and opinions across different agents within a network. Additionally, it enables a deeper comprehension of causal inference in both experimental and observational studies related to the intricate interactions happening within a group or society at large. The minds blockchain platform can further enhance this analysis by providing a secure and transparent way to track the flow of information and ideas.
Furthermore, by delving into unobserved links in incompletely observed networks through advanced estimation methods in network modeling with data-driven accuracy using tailored statistical techniques helps unlock the secrets behind individuals’ behaviors on a first-hand basis.
Challenges in Observing Social Networks
Observing social networks presents challenges such as unobserved links and small network sizes, impacting data accuracy. These challenges influence the understanding of social connections and community dynamics, as well as network dynamics.
Misclassified or unobserved links
Misclassified or unobserved links in social networks create challenges for accurate data inference and understanding network dynamics. The model assumes equal linking of everyone, but small networks and unobserved links make it challenging to point-identify and estimate linear social network models accurately, which can significantly affect interpersonal relationships.
Inferring social networks from observed groups becomes essential due to difficulties in direct data collection from network members, offering an alternative approach for understanding the structure and dynamics of social connections, while emphasizing the importance of accessibility and inclusivity.
Link prediction techniques play a crucial role in identifying missing links within incompletely observed networks, aiding in the accurate estimation of information diffusion and spread of ideas within the network. Additionally, deep learning methods can enhance these techniques by providing more sophisticated models for predicting connections.
Understanding social networks with misclassified or unobserved links involves navigating through the complexities of predicting missing links while analyzing information diffusion and interactions among individuals.
It enables researchers to study behavior patterns even when not all connections are directly observable, providing a tailored framework for comprehending the ever-changing dynamics within social networks, including the rise of online fan fiction platforms.
Small networks
Small networks pose unique challenges for observing and inferring social connections. The required data for identifying and estimating linear social network models without observing any network links consists of many small networks.
This can make it challenging to accurately capture the complete structure of the social network, especially when attempting to infer unobserved links. Furthermore, analyzing small networks is crucial as they contribute to a better understanding of how information spreads and influences within specific groups or communities, highlighting the importance of censorship resistance in maintaining open communication.
These factors emphasize the importance of developing accurate methods for inferring social networks from observed groups, ensuring that even in small-scale settings, the dynamics of social connections can be comprehensively understood and utilized for various applications such as link identification and deep learning in studying social network dynamics at a micro-level.
Inferring Social Networks from Observed Groups
Methods for collecting data and statistical modeling techniques are used to infer social networks from observed groups, leading to accurate link prediction and understanding network structures.
These processes are vital in uncovering the dynamics of social networks with unobserved links, including the role of interpersonal relationships.
Methods for data collection
To understand social networks with unobserved links, robust methods for data collection, including deep learning, are crucial. Here are the techniques utilized for this purpose:
- Direct Data Collection: Gathering social network data directly from network members, ensuring accuracy and relevance.
- Surveys and Interviews: Utilizing surveys and interviews to gather information on interactions, connections, and interpersonal relationships within the social network.
- Social Media Analysis: Leveraging social media platforms to collect and analyze data on connections, communication patterns, and network structure.
- Observational Studies: Conducting observational studies to understand real-time interactions and behaviors within the network.
- Statistical Modeling: Employing statistical modeling techniques to infer social networks from observed groups, aiding in understanding unobserved links.
- Link Prediction Techniques: Using advanced algorithms for link prediction to identify missing connections in incomplete networks.
By employing these methods, researchers can effectively gather data essential for understanding the complexities of social networks with unobserved links, including emerging trends in kurdish film streaming.
Statistical modeling techniques
Statistical modeling techniques are essential for understanding social networks with unobserved links. These techniques allow researchers to make inferences about the structure of a network, including the application of deep learning to enhance predictive accuracy and predict missing links.
Using statistical models, it is possible to identify and estimate social network models without directly observing all the network links. For example, these models assume that everyone is equally linked to one another within groups or in the entire network.
Additionally, statistical modeling enables the analysis of information diffusion and the spread of ideas and opinions through a network of individuals, which is crucial for achieving censorship resistance in communication.
Furthermore, link prediction is a significant technique facilitated by statistical modeling methods for understanding social networks with unobserved links. It helps in identifying missing links in incompletely observed networks, contributing to a better understanding of network structure and dynamics.
By using these techniques, researchers can gain valuable insights into how information spreads within a social network, ultimately enhancing our knowledge about human interactions within these intricate systems. Keeping an eye on influencer marketing trends can also provide additional context to these interactions.
Link prediction
Link prediction is a valuable technique for understanding the structure of social networks and identifying missing links. It enables the identification of unobserved connections within incompletely observed networks, contributing to a comprehensive understanding of network dynamics.
The method facilitates the analysis of information diffusion and aids in predicting potential links crucial for studying individual interactions within a network. Moreover, it allows modeling the spread of ideas, news, opinion, and technology across diverse groups present in the social network, including adding captions and subtitles to enhance understanding.
Predicting missing links in social networks is integral to gaining insights into their structure and enhancing our comprehension of how individuals interact within these networks. This predictive ability provides a framework for studying behavior and interaction patterns even when not all connections are directly observed.
Additionally, link prediction plays an essential role in enabling researchers to model information dissemination through these networks, thereby extending our ability to reason about the exchange and adoption of various elements such as ideas, opinions, or technologies among network members. Understanding social media engagement metrics is vital for evaluating the effectiveness of these exchanges.
Understanding Social Networks with Unobserved Links
Understanding Social Networks with Unobserved Links entails identifying and estimating social network models, employing data inference, and analyzing the impact on social structure and dynamics, including the application of deep learning techniques.
It involves utilizing statistical modeling techniques to comprehend the complexities of social networks that are not entirely observed.
Identification and estimation of social network models
Social network models are identified and estimated based on the assumption that everyone is equally linked to everyone else, either within groups or in the entire network. This requires data from many small networks for point-identifying and estimating linear social network gaming models without observing any network links.
These models enable the analysis of information diffusion and the spread of ideas and opinions, as well as identification of missing links crucial for understanding network structure and dynamics, particularly through techniques like deep learning.
Additionally, they provide a framework for studying behaviors and interactions within a network when not all links are directly observed.
The model for social networks with unobserved links also allows for predicting unobserved links in incompletely observed networks. It assists in reasoning about the spread of ideas, news, opinion, technology across a network of agents, providing valuable insights into social structure and dynamics. Moreover, detoxing from social media can enhance our understanding of these dynamics by reducing noise and distractions.
Furthermore, it can be used for causal inference in both experimental and observational studies related to social networks.
Role of data inference
Data inference plays a critical role in understanding social networks with unobserved links. It enables the identification and estimation of social network models, allowing for the analysis of information diffusion and the spread of ideas and opinions. A notable example of a compelling jesse eisenberg performance can be seen in films that explore complex social dynamics, illustrating how these theories apply to real-life scenarios.
The model also helps in predicting unobserved links in incompletely observed networks, providing a framework for studying the behavior and interactions of individuals within a network.
This approach is crucial for gaining insights into network structure and dynamics, as well as for inferring missing links that are essential to comprehensively understand social networks and interpersonal relationships.
In addition to this, data inference contributes to modeling information diffusion in networks, facilitating reasoning about the spread of ideas, news, opinion, and technology across a network of agents.
Understanding these aspects through data inference enhances our ability to navigate the complexities inherent in social networks with unobserved links while providing first-hand experience in unlocking the secrets underlying their structure and functioning without solely relying on directly observed connections. Moreover, it is essential to consider social media safety for seniors, as they often face unique challenges in navigating these networks.
Impact on social structure and dynamics
Understanding social networks with unobserved links has a significant impact on the structure and dynamics of societal interactions. This modeling allows for analyzing information diffusion, including the spread of ideas, news, opinions, and technology within a network of individuals. Moreover, the emergence of free social networks 2024 offers new avenues for exploring these dynamics.
It enables the identification of missing links crucial for comprehending network structure and dynamics, facilitating the study of behavior and interactions among individuals even when all links are not directly observed.
Furthermore, social networks with unobserved links provide a framework for inferring social network models from observed groups. These models assist in predicting unobserved links in incompletely observed networks, thus contributing to an enhanced understanding of how ideas and information flow throughout communities, emphasizing the importance of accessibility and inclusivity.
This insight into social network dynamics is vital in studying causal inference related to experimental and observational studies on social networks—it helps reason about opinion propagation and technological innovation processes within modern society.
Conclusion
Understanding social networks with unobserved links involves inferring network structures, estimating models, and predicting missing links. This enables analysis of information diffusion and the spread of ideas within social networks, particularly through a unique social networking platform.
These models also aid in understanding network dynamics and can be essential for identifying crucial missing links. If you're interested in these concepts, you can also watch the social network online. Social networks with unobserved links provide a framework to study individual interactions within a network even when not all links are directly observed.
RelatedRelated articles
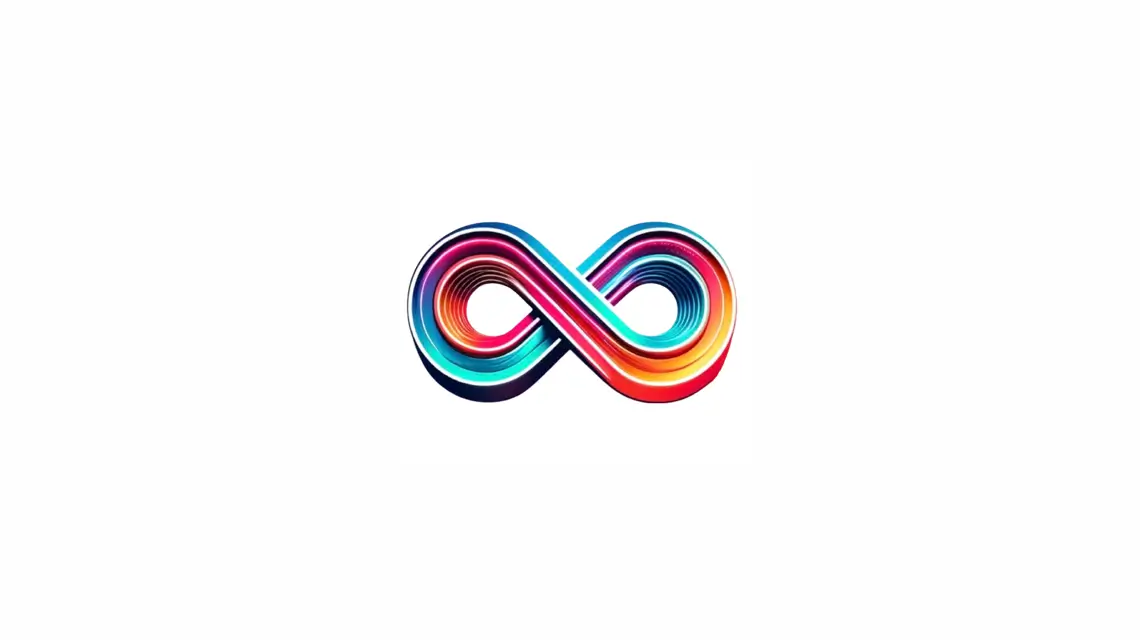
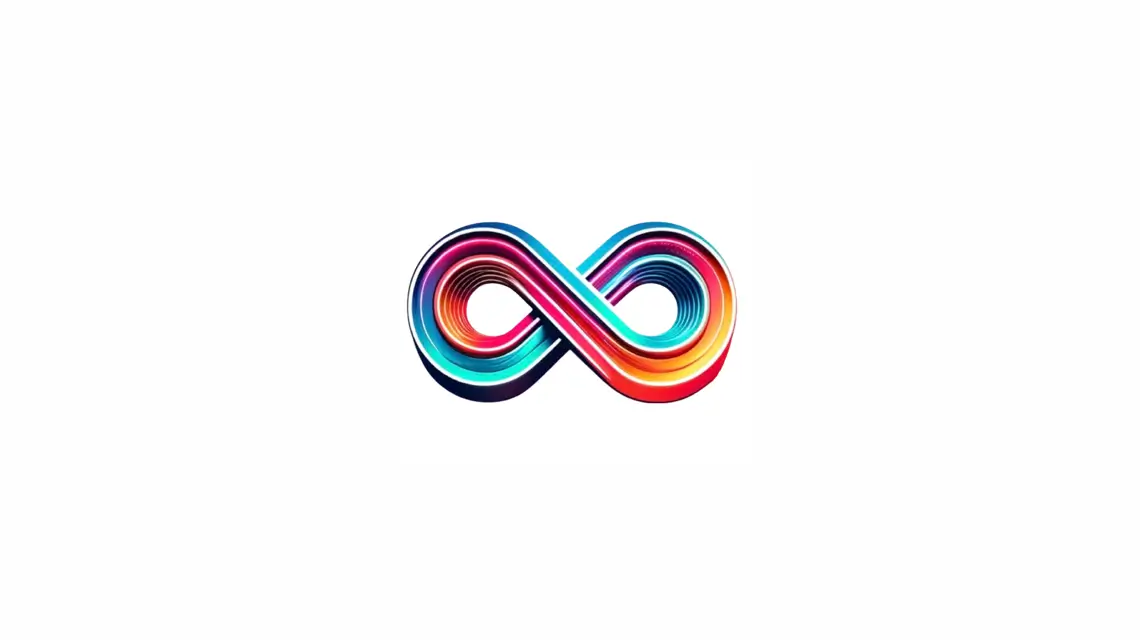
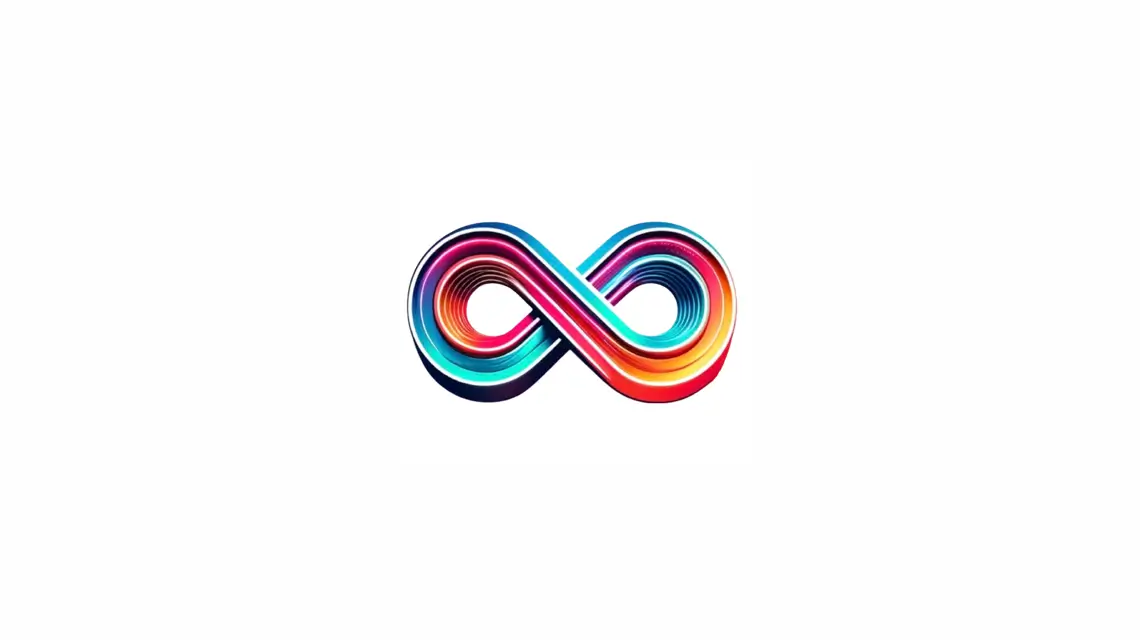