In today’s digital world, neural networks and social networks are key parts of our lives. They change how we get information, connect with others, and solve tough problems. These systems, like the human brain and social ties, are crucial for progress in artificial intelligence, machine learning, and analyzing networks.
Neural networks have nodes that work together like brain neurons. They process and send information when faced with a task or problem. Through complex math, they find the strongest connections in data to answer questions. This process, which is a key aspect of natural language processing, helps them handle many tasks, from recognizing images to understanding language.
Social networks show how people, groups, and organizations connect. They’re vital for sharing information, building communities, and predicting social actions. By studying these networks, experts and businesses can learn about human behavior, improve marketing, and forecast trends.
The link between neural networks and social networks opens new doors in artificial intelligence and machine learning. Deep neural networks find complex patterns in social data, including platforms like myspace music networking. This leads to better recommendation systems, sentiment analysis tools, and predictive models for many uses.
What is the Importance of Neural and Social Networks?
Neural networks are like the human brain in computers. They help computers learn and make decisions by spotting patterns in data. The more layers in a neural network, the deeper it gets. This lets experts create systems that can do things like recognize speech, identify images, and predict outcomes.
These networks are special because they can learn by themselves. They find patterns in different layers, which is crucial for tasks like community detection. This means computers can solve complex problems without needing to be told how. Artificial intelligence and machine learning rely on neural networks. They are key to making technology better.
Artificial Intelligence and Machine Learning
Artificial intelligence (AI) and machine learning (ML) go hand in hand with neural and social networks. AI uses ML to look at lots of data and learn from it. Machine learning is a part of AI that makes algorithms and models to help computers do tasks well.
Putting neural networks, AI, and ML together has changed many industries. It automates tasks, helps make better decisions, and brings new efficiency and innovation. Additionally, brand awareness campaigns have become more effective as these technologies grow, likely touching more parts of our lives.
Understanding Neural Network Architecture
Exploring neural network architecture helps us understand how artificial intelligence works, particularly through the lens of natural language processing. At the heart, we find three main layers: the input layer, the hidden layer(s), and the output layer. Each layer is crucial for processing and analyzing data to get the desired results.
Input, Hidden, and Output Layers
The input layer takes in data from the outside world. It sends this data to the next layers for deeper analysis. The hidden layer(s) then use complex algorithms to find deeper insights and patterns. Finally, the output layer shows the final result to the user.
Connections between layers, called weights, show how important each node is in the network. High weight values mean a big impact on the output. Low weights mean a smaller impact. Through training, neural networks get better at making decisions over time.
Knowing how neural networks work is key to using artificial intelligence and machine learning fully. By looking at the input, hidden, and output layers, we see how powerful these systems are, including their applications in natural language processing. They can uncover deep insights and help us make better decisions.
Deep Neural Networks and Deep Learning
Deep neural networks, or deep learning networks, are changing the game in artificial intelligence and machine learning. They have many hidden layers filled with artificial neurons that connect to each other. This makes them very complex.
These networks can learn and recognize complex patterns in data, making them particularly useful for community detection. They are great for tasks like computer vision, understanding human language, and predicting outcomes. They can turn any input into any output, but they need a lot of data to work well.
While simple machine learning models might use a few hundred examples, deep neural networks need millions. This lets them find even the smallest details in data. It opens up new possibilities in artificial intelligence.
Feature | Deep Neural Networks | Traditional Machine Learning |
---|---|---|
Architecture | Multi-layered with millions of interconnected neurons | Simpler, fewer layers and neurons |
Training Data | Requires millions of examples | Requires hundreds or thousands of examples |
Pattern Recognition | Excels at learning complex, nuanced patterns | Limited to simpler, more straightforward patterns |
Applications | Computer vision, natural language processing, predictive analytics | Narrower set of applications |
Deep neural networks are amazing at finding complex patterns in data. This makes them key to deep learning. As they get better, we’ll see more cool uses of artificial intelligence.
Social Networks and Community Dynamics
Social networks are like neural networks, with nodes and connections between them. In these networks, communities form with shared interests and values. They have their own political views, personal connections, and spiritual bonds. To truly connect with others, you must embrace your reality and understand the diverse perspectives that shape these communities.
What makes a community stand out includes feeling like you belong, having influence, and feeling fulfilled. Things like leadership, charm, and how often people join or leave can change a community’s power and structure.
Knowing how social networks work is key for many things, like sharing information and finding community groups. By understanding community dynamics, we can make better decisions and plan for the future. This helps us build strong community engagement. Additionally, it is essential to verify someone's identity to ensure trust and safety within these networks.
Key Elements of Community | Factors Impacting Community Dynamics |
---|---|
|
|
Applications in Network Analysis
The study of network analysis looks at how entities interact within a network. It uses neural and social networks to help with information diffusion, community detection, link prediction, and node embeddings. These methods are key in many areas, like social media and health studies.
Information diffusion shows how ideas spread through a network. Community detection finds groups of closely connected nodes. Link prediction helps guess new connections, which is useful for recommendations. Node embeddings turn nodes into vectors that show their place in the network.
These tools are used in many fields, from marketing to health. They help people make better decisions by showing hidden patterns. This leads to new ideas and improvements in many areas.
Application | Description | Potential Benefits |
---|---|---|
Information Diffusion | Modeling the spread of information, ideas, or behaviors through a network | Understand how trends and innovations propagate, enabling more effective marketing and communication strategies |
Community Detection | Identifying closely connected groups of nodes within a network | Gain insights into network structure and dynamics, inform targeted interventions and resource allocation |
Link Prediction | Forecasting new connections between nodes in a network | Improve recommendation systems, identify potential collaborations or partnerships |
Node Embeddings | Representing nodes as low-dimensional vectors that capture their structural properties | Enable a wide range of network-based applications, from anomaly detection to personalization |
Using network analysis, experts can find important insights and make smart choices. These methods help in many areas, from social media to health studies. They open up new chances for businesses and researchers.
Conclusion
The link between neural networks and social networks is key in today’s tech world. They’ve changed how we see artificial intelligence, machine learning, and network analysis. This has reshaped our view of the world.
Neural networks, like the human brain, have led to big leaps in artificial intelligence and machine learning, including advancements in natural language processing. These systems let computers learn, spot patterns, and make smart choices. This has changed many industries and how we use technology.
At the same time, social networks are vital for understanding how communities work and how we share information. The study of these networks has big impacts on tech, social sciences, and more. It’s changed how we talk, work together, and make choices in a world that’s more connected than ever.
RelatedRelated articles
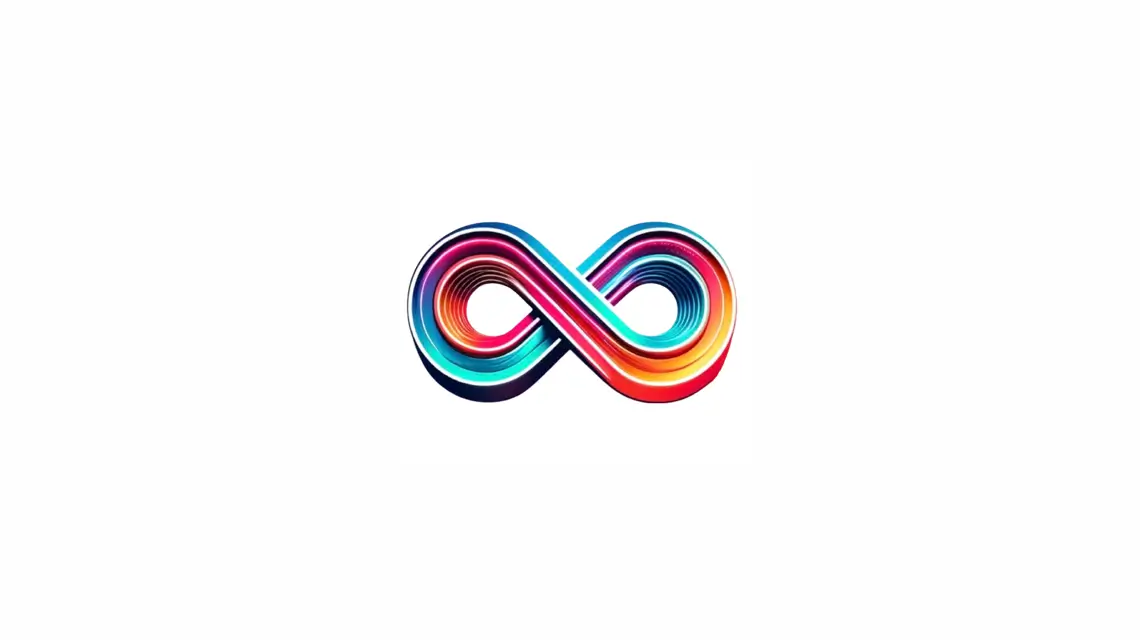
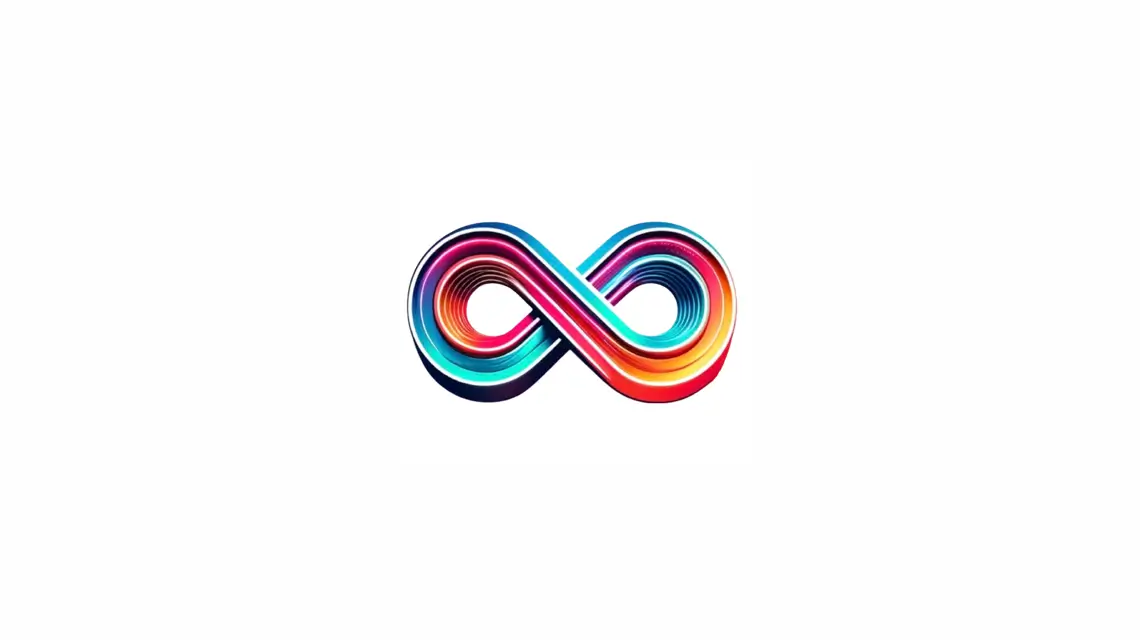
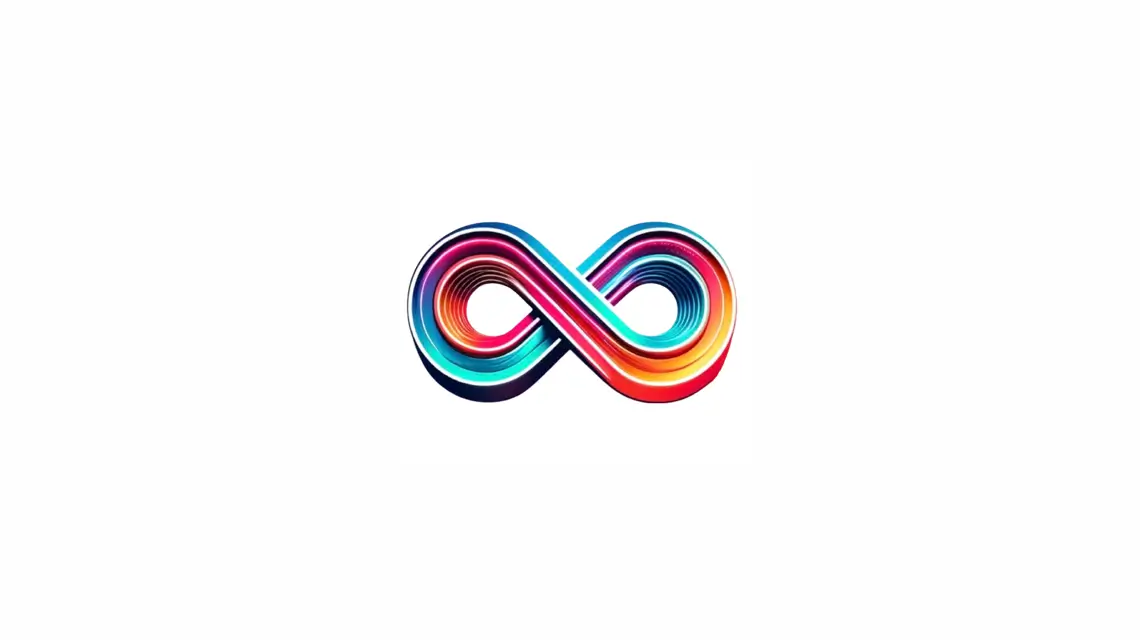